Data Driven and Model Supported Fusion of Multimodal Sensors
Data from real-life applications comprise several modalities that represent content with the same semantics from complementary aspects. Intraoperative tissue differentiation is also based on the fusion of considerable amounts of data with different information for the final assessment of the malignant potential of the respective target structure. The challenge is to enable the processing of multimodal data flows, i.e. the data recorded in real time by the sensor systems from projects A1-A5, for interventional diagnostics.
A novel feature fusion method based on a multi-level feature extraction structure is to be developed in order to fuse the various semantic feature groups and use the newly generated feature vectors for classification purposes.
Scientific Question
The multimodal measurement data, such as impedance and stiffness data, must be fused spatially and temporally as well as with the video data in order to be incorporated profitably into the tissue differentiation. Specific questions here are:
- How can data from different physical measurement principles of the electrical, mechanical and optical domains (A1-A5) be fused?
- How can measurements taken at different positions be incorporated into the sensor fusion?
- How can this increased complexity be used to expand tissue differentiation with regard to tumor type, grading and neoadjuvant therapy?
Various preliminary work from the first cohort can be used to address these questions. For example, the algorithms for landmark extraction (B1) and the developed Generative Adversarial Networks for depth estimation (B3) are used to localize the sensors. The database already designed provides the basis for an efficient data management system, which is to be developed in the second funding period.
Methodology and Solution Approaches
Data Driven Tissue Characterization
First, the sensor fusion is considered in reduced complexity by only taking into account sensor measurements of the same position. Feature vectors are extracted from the high-dimensional raw data of the sensor measurements in order to reduce the dimensionality of the data and transfer it into a common feature space. Multimodal autoencoders or transformer networks are suitable for this. The extracted latent feature representations can then be used to classify the tissue.
However, such approaches require large amounts of sensor data, which cannot be achieved with the available human tissue samples alone. One approach is to use the differentiation of animal tissue samples ex vivo to initialize a multimodal NN for sensor fusion. Subsequent augmentation of the data can further increase the amount of data available. In further steps, the pre-trained NN can be adapted to the intended tumor differentiation using real human test data and transfer learning approaches. Instead of relying on homogeneous, unimodal and labeled data sets as in conventional AI process workflows, transfer learning concepts can also be used to process realistic, multimodal and incomplete data sets.
Detection of Tumor Boundaries
In the intraoperative setup, it is not always possible or sensible to carry out measurements with different sensors at exactly the same position. In addition to the characterization of the tissue, the spread of the tumor and in particular the demarcation from healthy tissue is also essential. Therefore, the estimation of tumor boundaries is to be predicted on the basis of spatially distributed sensor measurements and coupled with the classification. The methods for intraoperative navigation developed in subproject B1 will be used for this purpose.
The camera image and the optical sensors (A1, A2) can be used, for example, to detect flat abnormalities and then provide initial information for a preliminary classification. The combined use of additional sensors for individual point measurements in border areas, e.g. with the WaFe sensor (A4) or the impedance sensor (A5), further narrows down the tumor area.
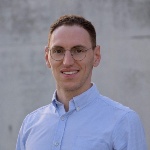
Matthias Ege
M.Sc.PhD Student B3
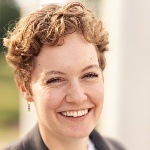
Carina Veil
Dr.-Ing.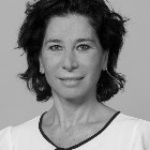
Cristina Tarín Sauer
Prof. Dr.-Ing.Principle Investigator of Subproject B3, Equal Opportunities Officer