Goals
- Establishment of standardized histological acquisition of tissue features on the preclinical model
- Development of a multiparametric phenotypic classifier of human tissue
- Validation of the classifier on human tumor tissue in direct comparison with sensor data
Work Program
Reproducible, quantitative evaluation strategies to develop an exact correlation of sensor data with the gold standard histology:
- Standardized classification of morphological parameters for direct comparison with sensor data
- Topographically exact quantification of vascularization, cell density, and fiber content
- Detection of tumor-induced stroma and vascular changes
- Development of a tool for standardized detection of iatrogenic tissue damage
- Multiparametric, topography-based tissue classification
- Multiparametric immunohistochemical tissue typing using image analysis
- Multiparametric immunoscore using multi-staining assays, RNAScope, and nanostring based expression analysis
- Validation of classifiers on human breast and bladder cancer samples
- Use of deep learning tools to link sensor and tissue data
Interactions with Other Projects
- with A1, A4, A5: Correlation of sensor data with tissue properties
- with A4, A5: Standardized detection of iatrogenic tissue damage
- with B1, B2: Use of machine learning methods for tissue classification
- with C1, C2: Validation of the multiparametric classifier on human tumor samples
Current Projects
Person in charge: cand. med. Saki Hassas
The main goal of the project is the development of an Artificial Intelligence-based image analysis algorithm, which can detect and classify non-invasive papillary urothelial carcinomas on routine stained, scanned histological sections. The algorithm is used to separate samples into papillary neoplasia with low malignant potential (PUNLMP), low-grade non-invasive papillary urothelial carcinoma, and high-grade non-invasive papillary urothelial carcinoma of the bladder. The classification of the algorithm takes into account various histological, immunohistochemical (p53, Mib-1 and CK-20), and molecular (p53 or FGFR mutations) criteria. Through the use of this technology, the establishment of an image-analytically supported diagnostic procedure for the differentiation of subgroups in urothelial carcinoma with different therapeutic approaches and prognoses can be accomplished.
Person in charge: cand. med. Diana Silimon
As part of group C3, this work is on the process of digitization of tissue slides of primary invasive ductal breast cancer (NST). The restriction to NST allows further investigations of differentiation and assessment of malignancy through Elston- Ellis Score, based on histological characteristics (tubule formation, nuclear topology, mitoses) and immunohistochemistry (ER, PR, HER2; Mib-1). An automated and reproducible method for detecting NST on tissue sections and quantifying biomarkers could reduce inter- and intraobserver variability and ensure a more efficient and consistent diagnostic workflow.
Editors
Massimo Granai
Dr. med.Clinician Scientist C3
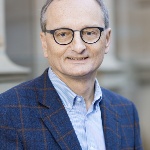
Falko Fend
Prof. Dr. med.Principle Investigator of Subproject C3