Multi-stain self-supervised learning on histopathological images
In order to effectively use different stainings in histopathological sections (e.g. H&E, estrogen, progesterone, HER2) and to transfer information between modalities, SSL methods are used and evaluated in cooperation with other subprojects. The aim is to create latent representations on individual modalities using SSL strategies which are then brought together, for example, through knowledge distillation or attention mechanisms. These representations are evaluated through various downstream tasks (classification, segmentation and object detection).
SSL for merging heterogeneous sources of image-based patient data
Extending the method for histopathological analysis, a higher-level processing chain for multimodal tissue classification is being developed based on general-purpose architectures, where the histopathological data and their latent representations serve as the basis for further image-based modalities. Through a unified latent space, findings from the pre- and postoperative analysis will be incorporated into the intraoperative diagnostics.
The prerequisite for this is also the collection of a patient-centered dataset using multiple image-based modalities from other subprojects. Thus, the development of an SQL-based platform for data acquisition will continue to include additional modalities and entities and yield a multi-purpose software.
Intraoperative visual tracking
For visual intraoperative tracking, a transformer-based approach is being developed that can robustly process longer video sections with several hundred frames. Publicly accessible data sets along with internal datasets are being used for training. Building on previous work and our own research on SSL strategies, new methods for tracking objects in the intraoperative environment are being developed.
3D reconstruction from intraoperative videos using NeRFs
In this subproject, the landmark-based methods from Project B1 are complemented by NeRF-based methods. To do this, videos of an intraoperative scene are first recorded in the JointLab in order to record the camera and light trajectory. This data is used to compare and evaluate different approaches. The methods developed for multi-view 3D reconstruction are finally evaluated in collaboration with other subgroups and tested on recorded scenes. This project leverages available positioning information to enable localization in low-texture environments.
Projektverantworliche
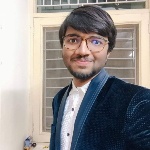
Valay Bundele
M.Sc.PhD Student B2
[Image: Valay Bundele]
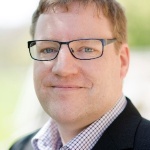
Hendrik Lensch
Prof. Dr.-Ing.Principle Investigator of Subproject B2
[Image: Hendrik Lensch, University of Tübingen ]