Efficient Spectroscopic Measurements due to In-situ Applications
A successful tumour resection is defined by its completeness. Nowadays, in most surgeries the tissue is analyzed using the so-called frozen section technique, where the material is cryogenically frozen, cut into thin slices and stained with haematoxylin eosin (HE) for instance. Afterwards, the specimen is investigated using a microscope where spectroscopic measurements can be taken as well (e.g. hyperspectral imaging using FTIR spectrometers). The complete investigation can last up to 30 minutes and the results of the staining are worse and harder to interpret than those of histopathological inspections, in which the tissue slices are embedded in formalin as well. Hence this research training group aims to get rid of those disadvantages tracing a multimodal approach in which several sensor types are being developed and examined. Those sensors shall enable in-situ investigations, which means that the measurements are undertaken within the living body during surgery. The multimodal approach shall increase the reliability of the sensors due to mutual validation.
Main problems using the frozen section technique:
- investigation time of approximately 30 minutes
- bad staining results
The approach in this subproject deals with hyperspectral imaging in the visible (VIS) and near-infrared (NIR) spectral range. The tissue is illuminated with polychromatic light and the spectral response of the tissue is detected two-dimensionally. The spectral and spatial information obtained in this way can be used to differentiate and classify different types of tissue on the basis of their spectrum.
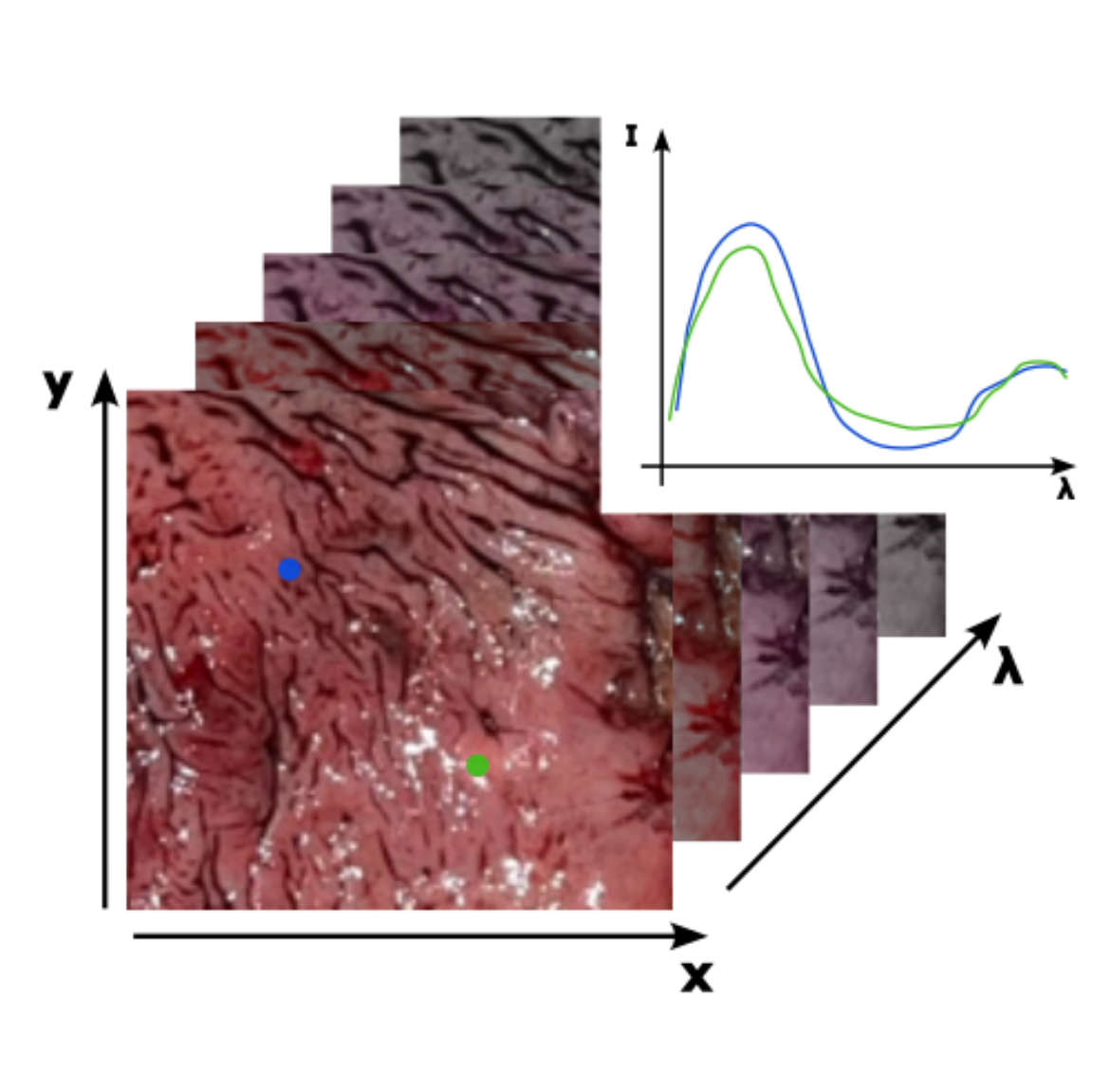
Optimized spectra
The results of the first PhD cohort have shown that a highly limited spectrum in the infrared can be sufficient to distinguish malignant from benign tissue. This will now be applied to the VIS/NIR spectral region and further developed towards more potent classification and optimization algorithms.
The use of a highly limited spectrum offers several advantages:
- better signal-to-noise ratio
- redction to the essential data
- shorter evaluation time
The first goal in this subproject is to find the best suited wavelengths to achieve the best distinguishing result. Therefore, the following approaches are examined:
- Development of a measurement setup with high spatial and spectral resolution for data generation.
- Data analysis using multivariate statistical methods (e.g. principal component analysis)
- Use of neural networks
Sensor Implementation into an Endoscope
Another challenge is to develop a miniaturized sensor so that it can be used endoscopically. Various hyperspectral snapshot systems are conceivable for this purpose, and their implementation must be evaluated in the course of the project. A central question will be how the optimized spectra can be realized.
Editors
Andrea Rüdinger
PhD Student A2
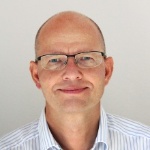
Stephan Reichelt
Prof. Dr.-Ing.Supervisor A1 and A2
- Profile page
- +49 711 685 66074
- Write e-mail
- Room: 1.234
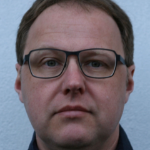
Tobias Haist
Dr.Supervisor of Subproject A1
- Profile page
- +49 711 685 66069
- Write e-mail
- Room: 1.253A